XYZ#5
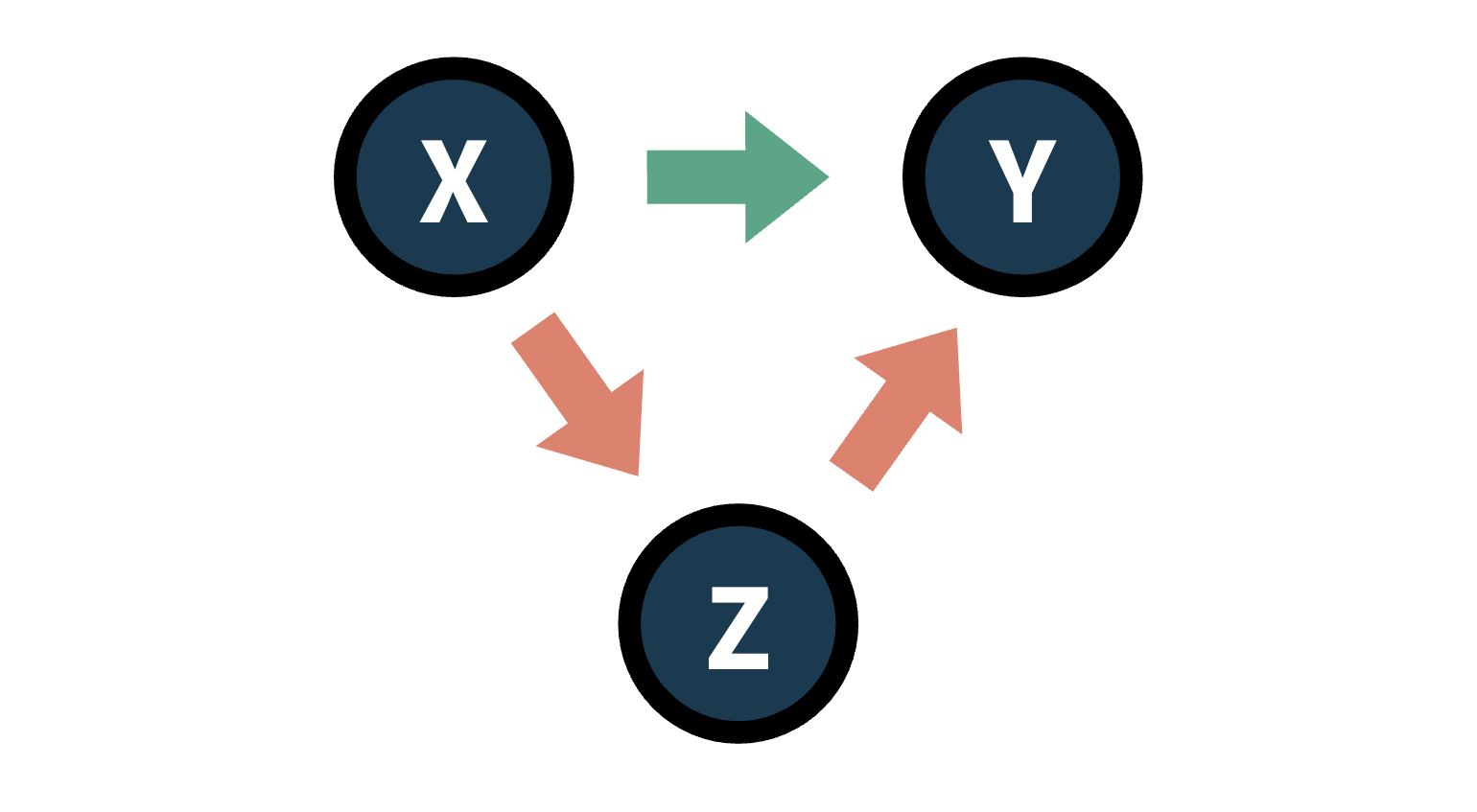
A causal inference newsletter
News
The Causal AI Conference 2023
Two weeks ago, CausalLens hosted one of the most important causal inference conferences, especially in the industry. The full conference is available on YouTube. The keynote speech by Guido Imbens talks about combining observational methods with experimental evidence (minute 33).
How Instacart Measures the True Value of Advertising
Instacart runs AB tests to measure the value of advertisement. The article underlines the importance to reduce dilution as much as possible, by using “ghost ads” to identify an appropriate control group. Moreover, Instacart is in the unique position, w.r.t. Google/Meta for example, to be able to both observe advertisement exposure and sales, which improves the precision of the data and ultimately of the estimates.
How to Accurately Test Significance with Difference in Difference Models
The article introduces Difference-in-Differences for settings in which it is not possible to run an AB test. The article presents 3 options to correct inference for time-series autocorrelation. The simplest option is averaging. However, we lose information and therefore power. The standard option is to cluster the standard errors at the unit level. The author’s preferred option is permutation testing: computing the treatment effect with many permuted treatment assignments and computing the p-value as the quantile of the estimated effect.
Old Reads
Factorial Designs, Model Selection, and (Incorrect) Inference in Randomized Experiments
The article studies experimental settings with multiple treatment arms and the trade-offs related to including all treatment interaction in the analysis. If the true interactions are zero, including them decreases the efficiency of the estimator. If they are not zero, the estimator is biased. The general recommendation is to always include all interactions in factorial designs.
Accelerating Online Experiments that Target Quantile Treatment Effects
When estimating quantile effects, variance reduction techniques result in biased estimates. This article covers an interesting technique to improve the efficiency of quantile treatment effect estimators that rewrites the quantile as an implicit function of the average treatment effect. This allow for CUPED-style variance reduction and unbiased estimates usind a method of moments estimator.
Encouragement Designs and Instrumental Variables for A/B Testing
Can you still do causal inference when you have to make the feature available to everyone? Yes, by encouraging some customers at random to use it. Encouragement designs provide unbiased estimates, but for a diffferent quantity: the average treatment effect for users for whom the encouragement works. The article also covers assumptions and inference in detail.
For more causal inference resources:
- Subscribe to my stories on Medium
- Follow me on LinkedIn
- Star
awesome-causal-inference
, where I collect all the articles and additional causal inference resources