XYZ#4
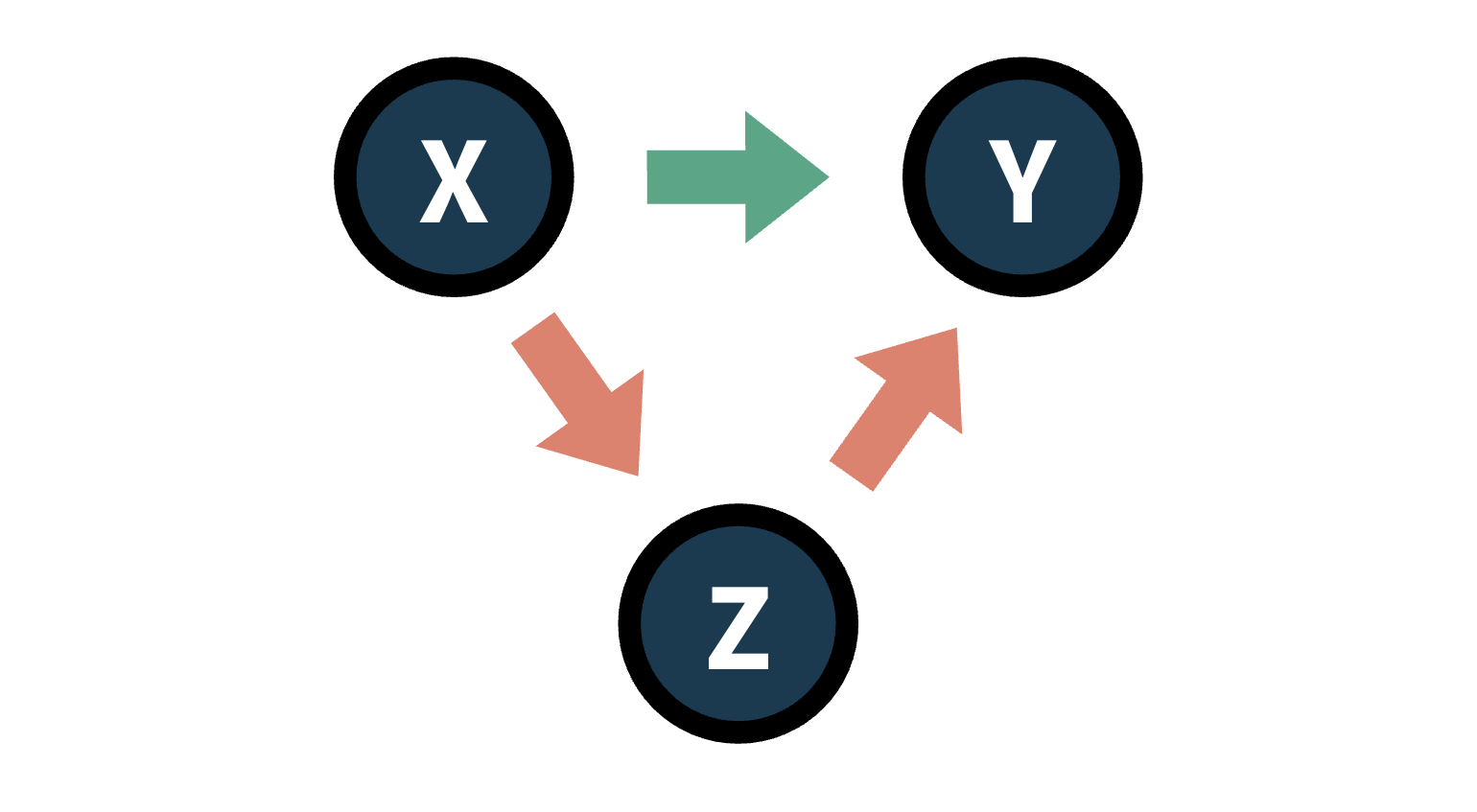
A causal inference newsletter
News
Encouragement Designs and Instrumental Variables for A/B Testing
Spotify researchers introduce IV for industry applications, when randomizing the treatment is not possible or desirable, for example because the feature is common knowledge. A trade-off that emerges in the industry is designing an encouragement that is sufficiently strong but does not violate the exclusion restriction.
Nixla Releases TimeGPT for Time Series Forecasting
TimeGPT isa generative model for time series trained over 100 billion rows of financial, weather, energy, and web data. It incorporates conformal prediction for uncertainty quantifications and should work with panel data (called multiple time series). Time series prediction is key in causal inference under unconfoundedness and for variance reduction.
Can I A/B Test That? Yes, with Few Exceptions
Ronny Kohavi shares practical advice on what to ask oneself when considering whether to test a feature. It also includes an insightful story from its time at Amazon, showing how testing constraints are usually not material harm but rather strong beliefs.
The Experimentation Platform Journey at Adevinta
Adevinta shares the story behind the evolution of their Experimentation Platform. At first it was just a Python package to unify AB testing in their Dutch market (Marktplaats), and then it evolved into a cross-marketplace AB testing platform, Houston.
Old Reads
The Balancing Act in Causal Inference
The propensity score is the unique balancing score, i.e. the unique function that balances any function of observables. However, in practice it needs to be estimated and inverted. The article compares inversity propensity weighting with different balancing approaches.
Using Balancing Weights to Target the Treatment Effect on the Treated when Overlap is Poor
The authors compare the performance of inverse propensity weighting (IPW) and balancing when overlap is limited. Across three sets of simulations they find that balancing produces unbiased results even with limited overlap, while IPW is biased.
Balancing Covariates via Propensity Score Weighting
IPW estimators can be very volatile because of propensity score inversion. The authors propose instead to weight by the probability of being assigned the opposite group. The estimator has the lowest asymptotic variance but the estimand, which they call “average treatment effect for the overlap population (ATO)”, is hard to interpret.
For more causal inference resources:
- Subscribe to my stories on Medium
- Follow me on LinkedIn
- Star
awesome-causal-inference
, where I collect all the articles and additional causal inference resources