XYZ#3
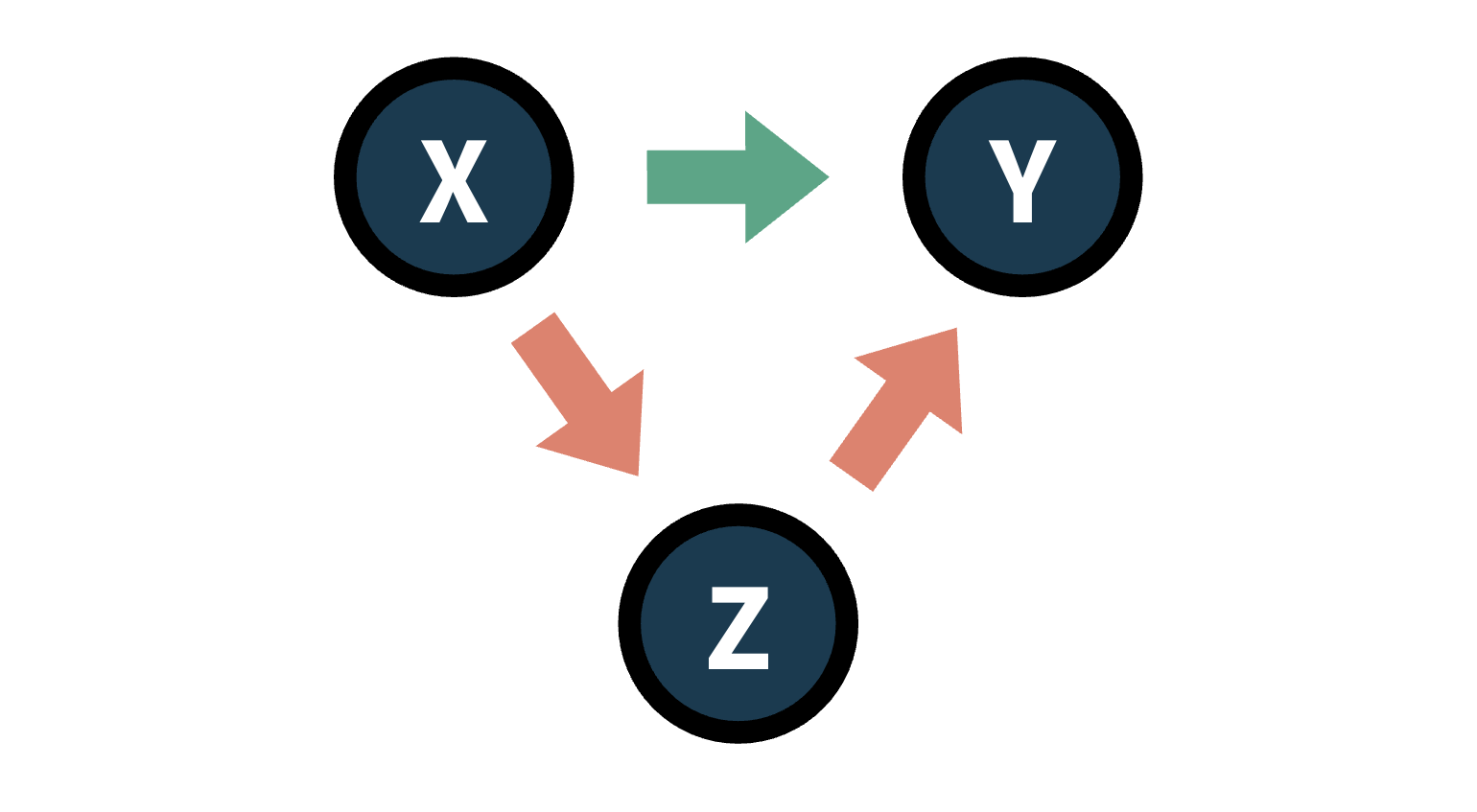
A causal inference newsletter
News
Speeding up HelloFresh’s Bayesian AB Testing
PyMC researchers tested different ideas to speed up thousands of concurrent AB tests. First, they decreased model parametrization, and sampling length, leading to marginal speed increases. However, the most gains were achieved by defining a single unpooled model made of many statistically independent models, leading to 60x speed gains.
A tidy
Package for HTE Estimation
A new R package, tidyhte
, estimates Heterogeneous Treatment Effects with a tidy syntax. The estimator is essentially an R-learner in the spirit of Kennedy (2022). It uses SuperLearner
for fitting nuisance parameters and vimp
for variable importance. Official website here.
Spotify Shares Some Experimentation Lessons
First, the researchers suggest thinking backward, starting from the decision that needs to be informed and designing the experiment accordingly. Second, they suggest running local experiments to discover local effects, an effective strategy for their expansion in the Japanese market. Last, they recommend testing changes incrementally and not in bunches.
Old Reads
Experimentation with Resource Constraints
Researchers at Stitchfix show how to deal with interference bias from budget constraints: enforce virtual constraints, preventing isolated groups from competing for resources. The solution is effective in solving the bias but opens new challenges in how to enforce the new constraints and scale the estimates to the full market.
R Guide for TMLE in Medical Research
This code-first guide introduces Targeted Maximum Likelihood Estimation (TMLE) through a medical application on real data: the effects of right heart catheterization on critically ill patients in the intensive care unit. The guide starts with data exploration, then introduces outcome models (G-computation), exposure models (IPW), and lastly combines them into TMLE.
An Overview of Netlix Experimentation Platform
Netflix’s experimentation platform is built on three pillars. The first pillar is a metrics repository where statistics are stored and shared across projects. The second pillar is a causal models library that collects causal inference methods. Lastly, a lightweight interactive visualization library to explore and report estimates. A global causal graph is not mentioned.
Thumbnack Explains the Efficiency Gains of Interleaving
First, interleaving is a one-sample test. Second, interleaving controls for customer variation since each customer sees both rankings. Third, signals are stronger since consumers have to make a choice (revealed preference). The downside is generalization since the rankings during the experiment differ from the deployed ones.
For more causal inference resources:
- Subscribe to my stories on Medium
- Follow me on LinkedIn
- Star
awesome-causal-inference
, where I collect all the articles and additional causal inference resources