XYZ#1
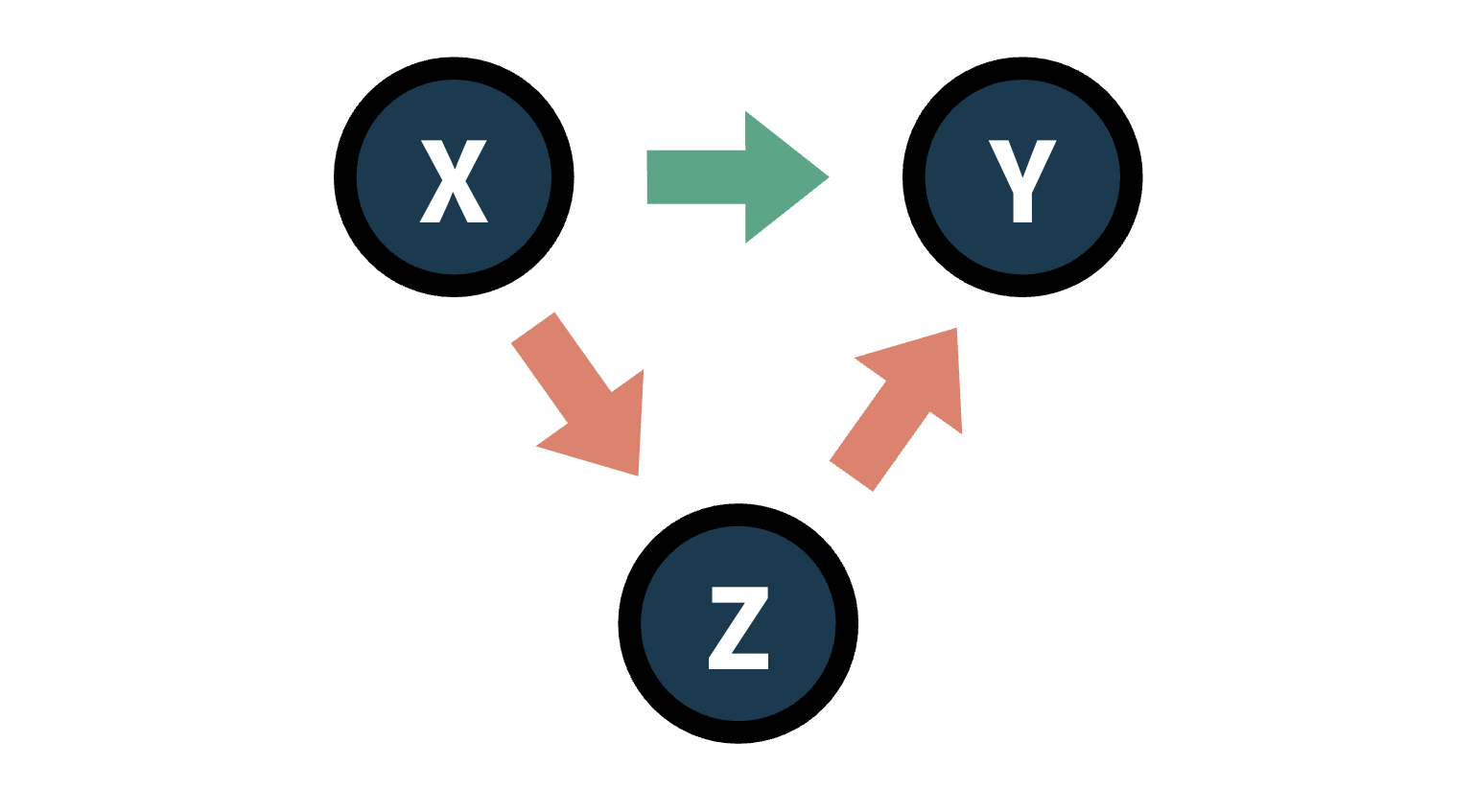
A causal inference newsletter
News
Spotify Releases its Experimentation Platform: Confidence
Spotify has released its internal experimentation platform, used for 10+ years to run and evaluate A/B tests at scale. Currently, it is in closed beta, but it promises large-scale performance, usability, flexibility, but also integration with existing A/B testing tools. A confidence
Github repo has been existing of a while.
Microsoft Calls for not Worrying About Interaction Effects
Microsoft researchers have looked at four major products, each running hundreds of A/B tests. They could not find any evidence of interaction effects. This does not mean interaction effects should be ignored, but rather that, at least in this scenario, they are of sufficiently low magnitude not to worry about them.
Updated Version of “Statistical Challenges in Online Controlled Experiments”
Ron Kohavi and co-authors have updated their paper on A/B test challenges, splitting the paper into a main paper and supplementary material. The main paper covers variance reduction, heterogeneous treatment effects, long-term effects, optimal stopping, and interference. The appendix covers stratified sampling, surrogate outcomes, and network A/B tests.
PyMC Adds the do-Operator
Python’s main probabilistic programming library has added a do-operator to do Bayesian Causal Analysis. If you know and specify the whole causal graph, it allows you to do Bayesian inference on causal quantities of interest. The repo is still experimental.
Old Reads
Embrace Overlapping A/B Tests and Avoid the Dangers of Isolating Experiments
From the experience of data scientists at Meta, strong interaction effects are rare. It’s worth isolating experiments only when it’s mechanically necessary, or they might break the user experience, or you need a very precise measurement.
An Analysts guide to MMM
While not being a standalone publication, this MMM guide in Meta’s Robyn documentation is comprehensive and detailed. It goes through all the phases of building an MMM model. Causality is mentioned shortly but vehemently: “we strongly recommend using experimental and causal results to calibrate MMM”.
An Intuitive Explanation of Why π is in the Normal Distribution
In two dimensions, the normal distribution can be defined by two properties: the pdf depends only on the distance from the origin, and the two dimensions are independent. From here, the relationship with a circle (and hence π) but also the relationship between the normal distribution and the Central Limit Theorem (covered in another video).
A List of Company Experimentation Platforms
Denise Visser lists the different in-house experimentation platforms of different companies as of 2020.
For more causal inference resources:
- Subscribe to my stories on Medium
- Follow me on LinkedIn
- Star
awesome-causal-inference